一、Hypothesis testing vs. estimation 假设检验和估计
- Estimation: Addresses the questions such as “what is this parameter’s value”. 主要用于估计总体参数的值。
- Hypothesis test:Addresses the questions such as “is the value of the parameter equal to a specific value”. 主要用于判断总体参数的某种假设是否成立。
二、Steps of hypothesis testing 假设检验的步骤
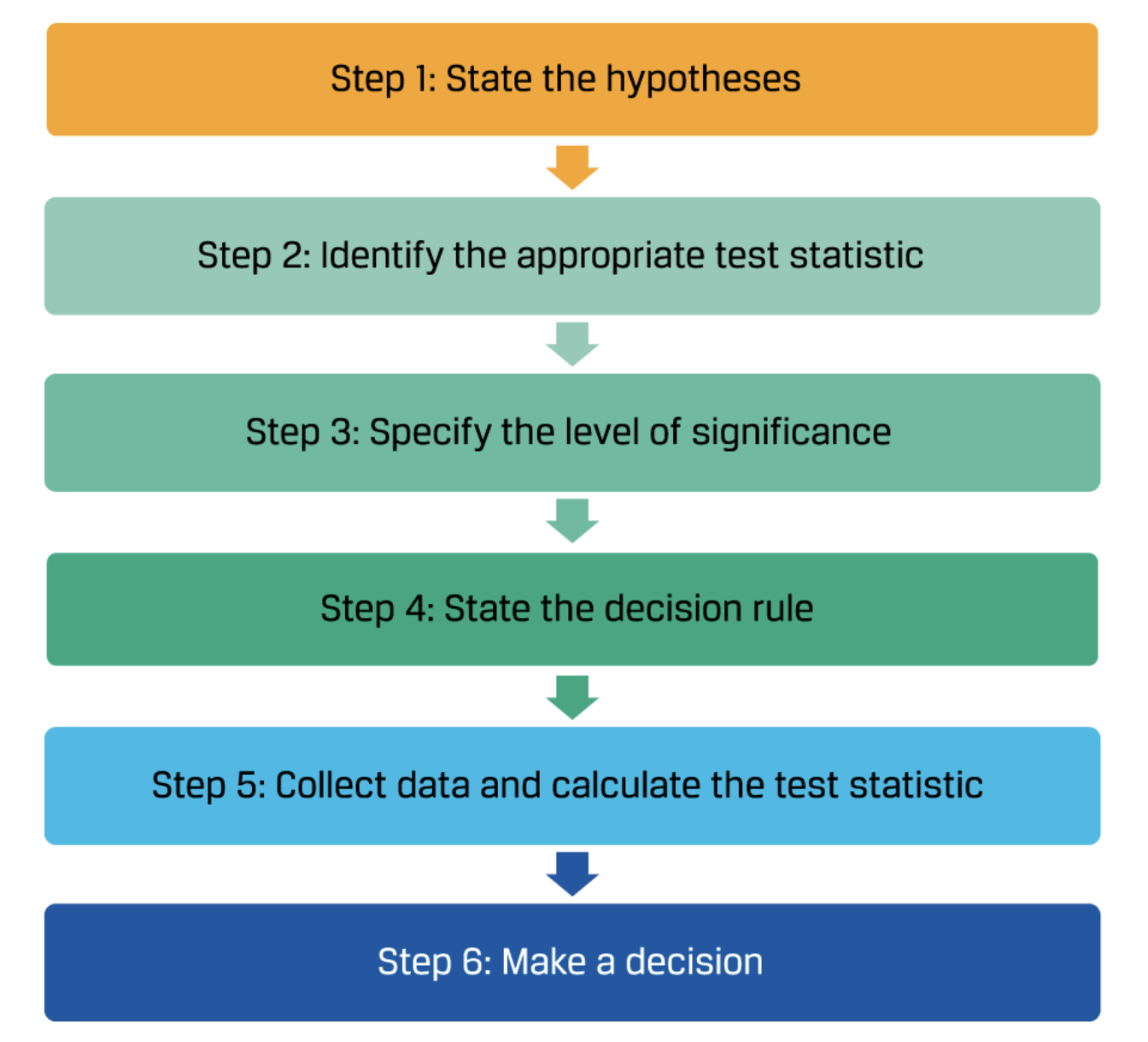
1. Stating the Hypotheses 给出假设
- Null hypothesis vs. Alternative hypothesis
- Null hypothesis 原假设:
- 定义:Hypothesis to be tested. 例如一支股票每个月的回报率等于1%。
- The “=” sigh will be only shown in null hypothesis. 只要带上等号,那么就是空假设。
- 空假设一般是分析师想去拒绝的;
- Alternative hypothesis 备择假设
- The opposite side of null hypothesis.
- 备择假设一般是分析师想去证明或者推断的。
- 如果原假设被拒绝,那么备择假设就被接受或者证明。
- Two-tailed test 双尾检验
- 我们想去检验一个population的均值是否等于6%;
- One-tailed test 单尾检验
- 我们想去检验一个population的均值是否大于6%
2. Identify the Appropriate Test Statistic 确定合适的检验统计量
- 检验统计量:一个检验统计量是由一个样本计算而来的量,其数值是我们决定是否拒绝原假设的一个基础。
- 我们统计角色关注的点在于检验统计量(test Statistics)的值。
- 检验统计量 = (样本统计量 - 在原假设下总体参数的值)/ 样本统计量的标准差
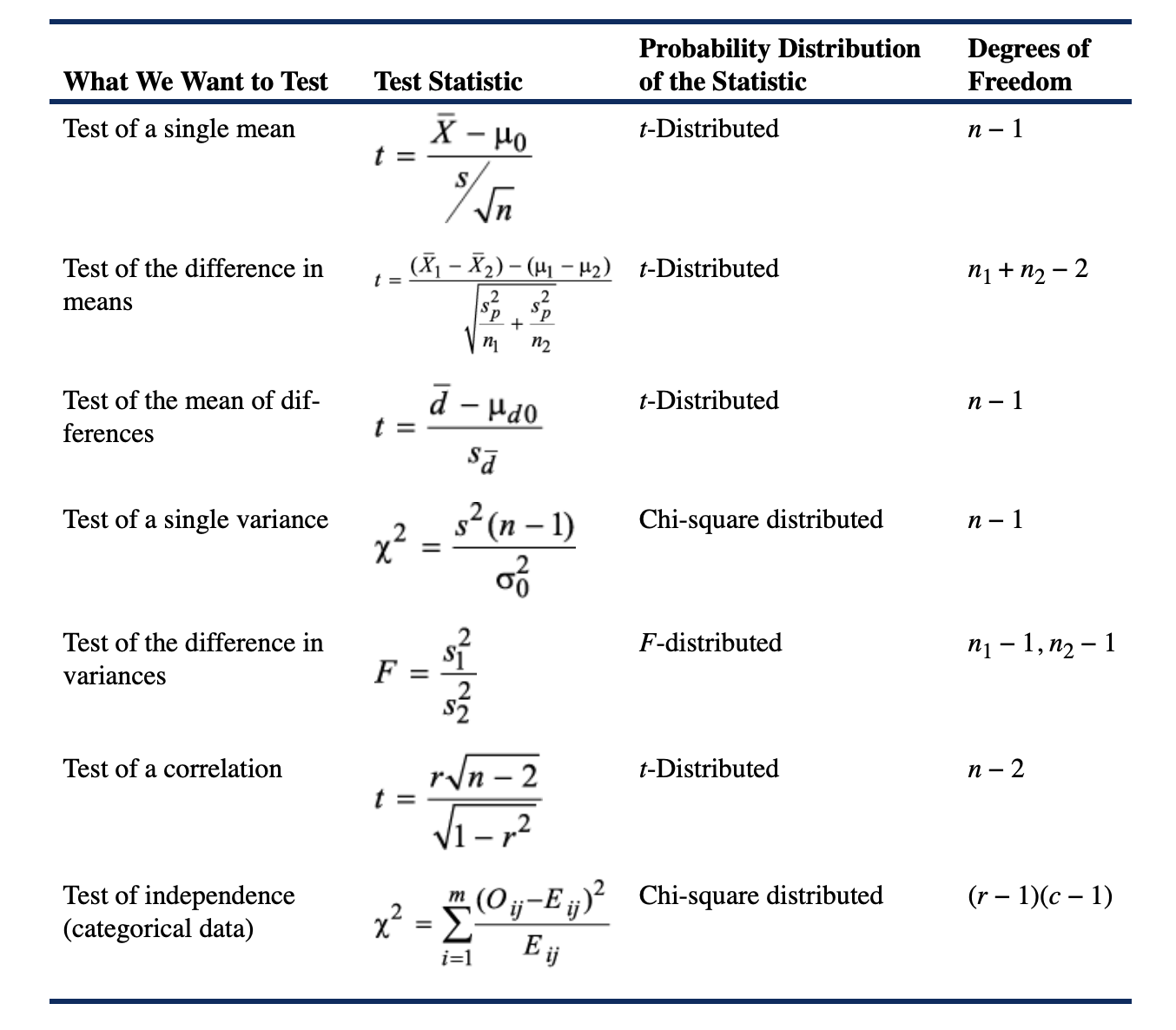
3. Specify the level of significance 指定显著性水平
- Significance level(σ) : 在假设检验中一类错误的概率记为希腊字母σ,这个概率被称为显著性水平。检验的一个0.05的显著性水平表示有5%的概率拒绝一个错误的原假设。
- confidence level (1-σ).
- “For example, a level of significance of 5% for a test means that there is a 5% probability of rejecting a true null hypothesis and corresponds to the 95% confidence level.”
- 我们在检验一个原假设时,有4种可能得结果:
- 拒绝一个错误的原假设,这是一个正确的决策;
- 我们拒绝一个正确的原假设,这被称为一类错误(type I error);
- 我们不能拒绝一个错误的原假设,这被称为二类错误(type II error);
- 我们不能拒绝一个正确的原假设,这是一个正确的决策;
- power of a test 检验的势: 正确拒绝原假设的概率,即在原假设为假时拒绝它的概率。
- 我们经常用来进行假设检验显著性水平有3个,分别为:0.10、0.05和0.01。如果我们在0.10的显著性水平拒绝了原假设,那么我们就具有了原假设为假的一些证据。如果我们在0.05的显著性水平下拒绝了原假设,那么我们就有了原假设为假的比较强的证据。如果我们在0.01的显著性水平下拒绝了原假设,那么我们就有原假设为假的相当强的证据。
4. State the decision rule 决策规则
- 检验统计量的拒绝点(rejection point)(临界值(critical value)的定义):一个检验统计量计算的拒绝点事与计算出来的检验统计量做比较的,以判断是否拒绝原假设的值;Rejection point是在显著性水平下的临界值。在双侧检验中,通常有两个拒绝点,分别位于分布的两端,使得拒绝域的总面积等于显著性水平(0.05)。
- P-value:the area in the probability distributions outside the calculated test statistic.The smallest level of significance at which the null hypothesis can be rejected. p-value 是一个概率值,表示观察到的统计量或更极端情况发生的概率,用于直接比较显著性水平。
- Decision rule
- p-value和level-of-confidence的区别
5. Collect data and calculate the test statistic
6. Make a decision
统计学上的显著性并不能代表经济学上的显著性,因为在实践中有交易成本、税、风险。
- Multiple tests(多重检验):是指在同一组数据集上进行多次假设检验的情况。在进行多重检验时,需要考虑到累积的错误率,以避免由于进行多次检验而增加犯错误的概率。在多重检验中,常见的问题是“多重比较问题”或“多重假设问题”,其中对同一组数据进行多次比较可能导致弃真错误(Type I Error)的概率增加。为了控制整体的错误率,通常采用一些校正方法,如Bonferroni校正、Holm校正等。
三、Testing on means,variance and correlation 关于均值、方差和相关系数的检验
- Test on mean
- Single mean
- z检验(方差已知)
- z是z分布;
是 sample mean 是总体均值的假定值 是总体的标准差
- t检验(方差未知)
是t分布 n-1 自由度 是 sample mean 是总体均值的假定值 - s是样本的标准差
- z检验(方差已知)
- Two means 对均值间差异的检验
- 原假设和备择假设
- t 检验
- Single mean
- Test on variance 对方差的假设检验
- Single variance
卡方检验 - Two variances F检验
- Single variance
- Test on correlation 相关性检验
- Parametric test
- Test on Pearson correlation,
,df = n-2
- Test on Pearson correlation,
- Nonparametric test
- Test on Spearman correlation,
- Test on Spearman correlation,
- Parametric test